I work in Sequential Decision Analytics, focusing on problems with high-dimensional, discrete decision spaces from Operations Research. To solve those problems, I apply Stochastic Programming and Reinforcement Learning. I apply my knowledge to Healthcare Logistics problems such as capacity allocation during infectious outbreaks and scheduling of surgeries or appointments.
Expertise
Nursing and Health Professions
- Time
- Patient
- Hospital
Computer Science
- Heuristics
- Linear Program
- Programs
- Service
- queueing model
Organisations
Publications
Research profiles
I am responsible for the Master course on Reinforcement Learning with a special focus on the ODE approach of Stochastic Approximation and how it can be applied to show the convergence of Reinforcement Learning algorithms.
In addition, I supervise Bachelor, Master and Internship students, mostly working on topics within Healthcare Logistics.
Affiliated study programs
Courses academic year 2023/2024
Courses in the current academic year are added at the moment they are finalised in the Osiris system. Therefore it is possible that the list is not yet complete for the whole academic year.
Courses academic year 2022/2023
Current projects
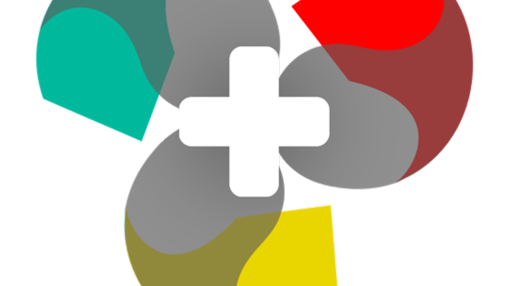
Regular and Unplanned Care Adaptive Dashboard for Cross-Border Emergencies
During cross-border health emergencies, health and care services may be overwhelmed by high numbers of patients requiring unplanned care. Delays and backlogs in regular care, as a result of the stretched healthcare system, leave millions of patients with regular care needs unattended, resulting in disastrous healthcare outcomes. If poor healthcare outcomes across the population are to be avoided, healthcare systems must become more resilient and flexible and allow for rapid changes in the care delivery services. RAPIDE aims to develop, validate, and demonstrate a portfolio of powerful tools that enable healthcare systems to increase the robustness of decisions, the resilience of healthcare professionals and patients, and the flexibility in the modalities of care delivery, thereby maintaining access to regular care during health emergencies. RAPIDE emphasizes opportunities for optimizing in-hospital care but also for relocating care from hospitals to community and home environments without loss of care quality. Thus, the project focuses on two closely linked challenges – 1: Identifying and predicting how much care and which care needs to be moved along the care chain; 2: Identifying and verifying effective, feasible, and acceptable ways to make this reconfiguration of care a reality. This will be achieved by (a) resource modeling, which builds comprehensive foresight and forecasting solutions and links them to patient flow optimization along the whole care chain, and (b) selecting and implementing the best available tools to deliver regular care in new ways. RAPIDE will be co-designed and co-validated with stakeholders, from patients, GPs, clinicians, and hospital managers to health ministries, pandemic management, and public health agencies, to ensure usability, acceptability, and equitable real-world value.
Supporting Efficient Deployment of Nursing Home Staff Through Demand Prediction
Hospitals are discharging many patients that are in need of aftercare, e.g., requiring a bed in a nursing home. The timely transfer of those patients to the correct type of aftercare is of utmost importance to ensure the quality of care, i.e., that the patient receives the right treatment at the right time, as well as the quality of staffing, i.e., that the capacity and staff in the hospitals and the aftercare organizations are used efficiently. If patients do not receive timely treatment, it may negatively impact their recovery journey. Further, they stay in the hospital bed longer than necessary, producing so-called bed-blocking days. These blocked beds are not available to other patients in need, and highly trained and costly staff needs to take care of those patients instead of performing tasks corresponding to their skill set. This project focuses on developing models to predict demand for nursing home care originating from hospitals on different time scales (long-term, mid-term, and short-term). Those hospital predictions will be made available to nearby nursing homes in order to support their capacity and staff planning. By accurately predicting demand and aligning resources accordingly, the project seeks to reduce bed-blocking, improve patient outcomes, and enhance the efficiency of staff planning in nursing homes and hospitals. The project involves collaboration with multiple hospitals to ensure its applicability and aims to develop a prototype prediction tool for immediate use, with the long-term goal of integrating the models into hospital information systems for widespread implementation.
In the press
Address
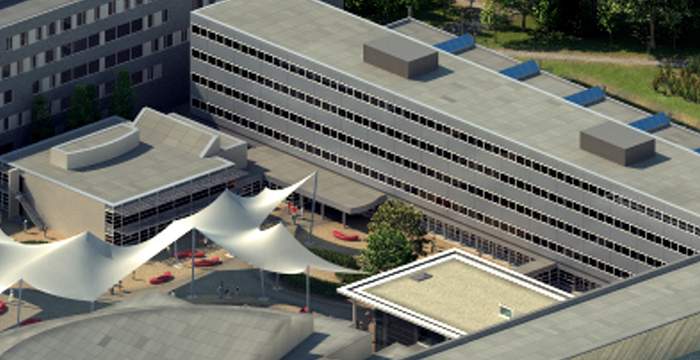
University of Twente
Zilverling (building no. 11), room 4031
Hallenweg 19
7522 NH Enschede
Netherlands
University of Twente
Zilverling 4031
P.O. Box 217
7500 AE Enschede
Netherlands