Since 2018, Johannes Schmidt-Hieber is professor of statistics at the University of Twente. Click here here to access the personal webpage.
Expertise
Mathematics
- Gaussian Process
- Bounds
- Kriging
- Parameters
- Bayesian
Economics, Econometrics and Finance
- Measure of Dispersion
- Estimation Theory
- Information
Organisations
Articles
- Bayesian variance estimation in the Gaussian sequence model with partial information on the means preprint
With Gianluca Finocchio. - Posterior consistency for n in the binomial (n,p) problem with both parameters unknown - with applications to quantitative nanoscopy. preprint
With Laura Schneider, Thomas Staudt, Andrea Krajina, Timo Aspelmeier and Axel Munk. - Posterior contraction rates for support boundary recovery preprint
With Markus Reiss. - Nonparametric regression using deep neural networks with ReLU activation function preprint
To appear as a discussion article in the Annals of Statistics - Tests for qualitative features in the random coefficients model preprint
With Fabian Dunker, Konstantin Eckle, and Katharina Proksch.
To appear in Electronic Journal of Statistics - Nonparametric Bayesian analysis of the compound Poisson prior for support boundary recovery preprint
With Markus Reiss.
To appear in Annals of Statistics - Asymptotic nonequivalence of density estimation and Gaussian white noise for small densities preprint
With Kolyan Ray.
To appear in Annales de l'Institut Henri Poincaré B. - A comparison of deep networks with ReLU activation function and linear spline-type methods pdf
Neural Networks, Volume 110, 232-242, 2019.
With Konstantin Eckle. - The Le Cam distance between density estimation, Poisson processes and Gaussian white noise article preprint
Mathematical Statistics and Learning. Volume 1, Issue 2, 101-170, 2018. With Kolyan Ray. - A regularity class for the roots of non-negative functions. pdf arXiv
Annali di Matematica Pura ed Applicata. Volume 196, Number 6, 2091-2103, 2017. With Kolyan Ray. - Minimax theory for a class of non-linear statistical inverse problems. article revised preprint
Inverse Problems. Volume 32, Number 6, 065003, 2016. With Kolyan Ray. - Conditions for posterior contraction in the sparse normal means problem. pdf
Electronic Journal of Statistics. Volume 10, Number 1, 976-1000, 2016. With Stéphanie van der Pas and JB Salomond. - Bayesian linear regression with sparse priors. pdf arXiv
Annals of Statistics. Volume 43, Number 5, 1986-2018, 2015. With Ismael Castillo and Aad van der Vaart. - On adaptive posterior concentration rates. pdf
Annals of Statistics. Volume 43, Number 5, 2259-2295, 2015. With Marc Hoffmann and Judith Rousseau. - Spot volatility estimation for high-frequency data: adaptive estimation in practice. pdf arXiv
Springer Lecture Notes in Statistics: Modeling and Stochastic Learning for Forecasting in High Dimension. 213-241, 2015. With Till Sabel and Axel Munk. - Asymptotic equivalence for regression under fractional noise. pdf arXiv
Annals of Statistics, Volume 42, Number 6, 2557-2585, 2014. - Asymptotically efficient estimation of a scale parameter in Gaussian time series and closed-form expressions for the Fisher information. pdf arXiv supplement
Bernoulli, Volume 20, Number 2, 747-774, 2014. With Till Sabel. - On an estimator achieving the adaptive rate in nonparametric regression under Lp-loss for all 1≤p≤∞. preprint
This is an update of the working paper pdf. In the first version, we only consider simultaneous adaptation with respect to L2- and L∞-loss. This article might be easier to read and includes a small numerical study. - Multiscale methods for shape constraints in deconvolution: Confidence statements for qualitative features.pdfsupplement
Annals of Statistics, Volume 41, Number 3, 1299-1328, 2013. With Axel Munk and Lutz Dümbgen.
A first draft of this paper appeared under the title: "Multiscale methods for shape constraints in deconvolution" in 2011.pdf. It contains essentially the same results, but under a very strong assumption on the decay of the Fourier transform of the error density. The first version is much easier to read and does not require the theory of pseudo-differential operators. - Adaptive wavelet estimation of the diffusion coefficient under additive error measurements. pdf software
Annales de l'Institut Henri Poincaré, 48, 1186-1216. With Marc Hoffmann and Axel Munk.
An earlier version of this paper was published as a working paper under the title "Nonparametric estimation of the volatility under microstructure noise: wavelet adaptation." pdf. - Nonparametric methods in spot volatility estimation. pdf
Dissertation. Universität Göttingen und Universtät Bern, 2010. - Lower bounds for volatility estimation in microstructure noise models. pdf
Borrowing Strength: Theory Powering Applications - A Festschrift for Lawrence D. Brown, IMS Collections, 6, 43-55, 2010. With Axel Munk. - Nonparametric estimation of the volatility function in a high-frequency model corrupted by noise. pdf
Electronic Journal of Statistics, 4, 781-821, 2010. With Axel Munk. - Sharp minimax estimation of the variance of Brownian motion corrupted with Gaussian noise. pdf (including supplementary material).
Statistica Sinica, 20, 1011-1024, 2010 . With T. Tony Cai and Axel Munk.
Publications
Jump to: 2025 | 2024 | 2023 | 2022 | 2021
2025
Transfer learning, generative modelling, and nonparametric regression (2025)[Thesis › PhD Thesis - Research UT, graduation UT]. University of Twente. Zamolodtchikov, P.https://doi.org/10.3990/1.9789036564649
2024
Convergence guarantees for forward gradient descent in the linear regression model (2024)Journal of statistical planning and inference, 233. Article 106174. Bos, T. & Schmidt-Hieber, J.https://doi.org/10.1016/j.jspi.2024.106174Improving the Convergence Rates of Forward Gradient Descent with Repeated Sampling (2024)[Working paper › Preprint]. ArXiv.org. Dexheimer, N. & Schmidt-Hieber, J.https://doi.org/10.48550/arXiv.2411.17567Understanding the Effect of GCN Convolutions in Regression Tasks (2024)[Working paper › Preprint]. ArXiv.org. Chen, J., Schmidt-Hieber, J., Donnat, C. & Klopp, O.https://doi.org/10.48550/arXiv.2410.20068On the VC dimension of deep group convolutional neural networks (2024)[Working paper › Preprint]. ArXiv.org. Sepliarskaia, A., Langer, S. & Schmidt-Hieber, J.https://doi.org/10.48550/arXiv.2410.15800Lower bounds for the trade-off between bias and mean absolute deviation (2024)Statistics & probability letters, 213. Article 110182. Derumigny, A. & Schmidt-Hieber, J.https://doi.org/10.1016/j.spl.2024.110182Local convergence rates of the nonparametric least squares estimator with applications to transfer learning (2024)Bernoulli, 30(3), 1845-1877. Schmidt-Hieber, J. & Zamolodtchikov, P.https://doi.org/10.3150/23-BEJ1655Dropout Regularization Versus l2-Penalization in the Linear Model (2024)Journal of machine learning research, 25, 1-48. Article 204. Clara, G., Langer, S. & Schmidt-Hieber, J.https://www.jmlr.org/papers/v25/23-0803.htmlCodivergences and information matrices (2024)Information Geometry, 7, 253-282. Derumigny, A. & Schmidt-Hieber, J.https://doi.org/10.1007/s41884-024-00135-2On the inability of Gaussian process regression to optimally learn compositional functions (2024)In NIPS'22: Proceedings of the 36th International Conference on Neural Information Processing Systems (pp. 22341 -22353). Article 1623. Curran Associates Inc.. Giordano, M., Ray, K. & Schmidt-Hieber, J.https://doi.org/10.5555/3600270Johannes Schmidt-Hieber's contribution to the Discussion of 'the Discussion Meeting on Probabilistic and statistical aspects of machine learning' (2024)Journal of the Royal Statistical Society. Series B: Statistical Methodology, 86(2), 329. Schmidt-Hieber, J.https://doi.org/10.1093/jrsssb/qkae007Correction to “Nonparametric regression using deep neural networks with ReLU activation function” (2024)Annals of statistics, 52(1), 413-414. Schmidt-Hieber, J. & Vu, D.https://doi.org/10.1214/24-AOS2351A supervised deep learning method for nonparametric density estimation (2024)Electronic Journal of Statistics, 18(2), 5601-5658. Bos, T. & Schmidt-Hieber, J.https://doi.org/10.1214/24-EJS2332
2023
Hebbian learning inspired estimation of the linear regression parameters from queries (2023)[Working paper › Preprint]. ArXiv.org. Schmidt-Hieber, A. J. & Koolen, W. M.https://doi.org/10.48550/arXiv.2311.03483On lower bounds for the bias-variance trade-off (2023)Annals of the Institute of Statistical Mathematics, 51(4), 1510 - 1533. Derumigny, A. & Schmidt-Hieber, A. J.https://doi.org/10.1214/23-AOS2279Lower bounds for the trade-off between bias and mean absolute deviation (2023)[Working paper › Preprint]. ArXiv.org. Derumigny, A. & Schmidt-Hieber, J.https://doi.org/10.48550/arXiv.2303.11706On Generalization Bounds for Deep Networks Based on Loss Surface Implicit Regularization (2023)IEEE transactions on information theory, 69(2), 1203- 1223. Imaizumi, M. & Schmidt-Hieber, A. J.https://doi.org/10.1109/TIT.2022.3215088Posterior Contraction for Deep Gaussian Process Priors (2023)Journal of machine learning research, 24(66), 1-49. Finocchio, G. & Schmidt-Hieber, A. J.https://jmlr.org/papers/volume24/21-0556/21-0556.pdf
2022
On the inability of Gaussian process regression to optimally learn compositional functions (2022)[Working paper › Preprint]. ArXiv.org. Giordano, M., Ray, K. & Schmidt-Hieber, J.https://doi.org/10.48550/arXiv.2205.07764Local convergence rates of the least squares estimator with applications to transfer learning (2022)[Working paper › Preprint]. ArXiv.org. Schmidt-Hieber, J. & Zamolodtchikov, P.https://doi.org/10.48550/arXiv.2204.05003Convergence rates of deep ReLU networks for multiclass classification (2022)Electronic Journal of Statistics, 16(1), 2724 - 2773. Bos, T. & Schmidt-Hieber, A. J.https://doi.org/10.1214/22-EJS2011On the inability of Gaussian process regression to optimally learn compositional functions (2022)In 36th Conference on Neural Information Processing Systems, NeurIPS 2022 (Advances in Neural Information Processing Systems; Vol. 35). Neural information processing systems foundation. Giordano, M., Ray, K. & Schmidt-Hieber, J.https://papers.nips.cc/paper_files/paper/2022/hash/8c420176b45e923cf99dee1d7356a763-Abstract-Conference.html
2021
Posterior analysis of n in the binomial (n,p) problem with both parameters unknown—with applications to quantitative nanoscopy (2021)Annals of statistics, 49(6), 3534-3558. Schmidt-Hieber, A. J., Schneider, L., Staudt, T., Krajina, A., Aspelmeier, T. & Munk, A.https://doi.org/10.1214/21-AOS2096Two perspectives on high-dimensional estimation problems: posterior contraction and median-of-means (2021)[Thesis › PhD Thesis - Research UT, graduation UT]. University of Twente. Finocchio, G.https://doi.org/10.3990/1.9789036552356Posterior contraction for deep Gaussian process priors (2021)[Working paper › Working paper]. Finocchio, G. & Schmidt-Hieber, A. J.https://arxiv.org/abs/2105.07410
Research profiles
Courses academic year 2024/2025
Courses in the current academic year are added at the moment they are finalised in the Osiris system. Therefore it is possible that the list is not yet complete for the whole academic year.
- 191508209 - Internship AM
- 191508309 - Final Project (combination)
- 191508409 - Final Project M-AM
- 202001350 - Analysis II
- 202100112 - Graphical Models and Causality
- 202300016 - Mathematical Statistics 1
- 202300017 - Analysis 3
- 202300018 - Reflection 1
- 202300026 - Mathematical Statistics 2
- 202300130 - Capita Selecta Applied Mathematics
- 202400606 - Statistical Learning
- 202400669 - Capita Selecta Applied Mathematics 2
Courses academic year 2023/2024
- 191508209 - Internship AM
- 191508309 - Final Project (combination)
- 191508409 - Final Project M-AM
- 200900030 - Research of Math
- 201900115 - Statistical Learning
- 202001348 - Mathematical Statistics
- 202001349 - Project Statistics
- 202001350 - Analysis II
- 202001351 - Prooflab Revisited: Diversity in Culture
- 202001385 - Bachelor Assignment AM-TCS Double Degree
- 202100112 - Graphical Models and Causality
- 202200398 - Internship AM-CS
- 202300016 - Mathematical Statistics 1
- 202300017 - Analysis 3
- 202300018 - Reflection 1
- 202300026 - Mathematical Statistics 2
- 202300130 - Capita Selecta Applied Mathematics
Address
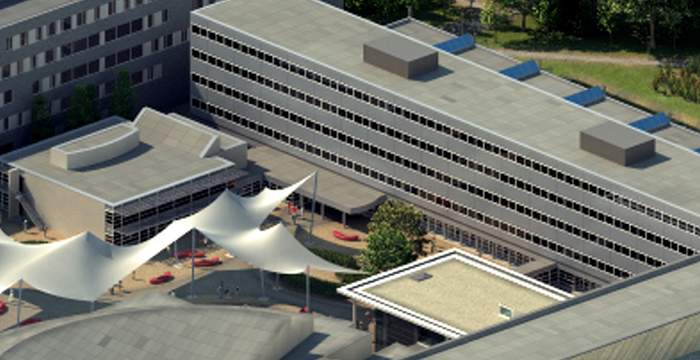
University of Twente
Zilverling (building no. 11), room 2057
Hallenweg 19
7522 NH Enschede
Netherlands
University of Twente
Zilverling 2057
P.O. Box 217
7500 AE Enschede
Netherlands