I obtained my PhD in 2021 at Paris Brain Institute with a thesis entitled Reproducible and interpretable deep learning for the diagnosis, prognosis and subtyping of Alzheimerâs disease from neuroimaging data. Another main output of my PhD is the contributions to two Python libraries:
My research interests are in deep learning for medical image analysis, in particular the study of their validity and robustness and their application to neurological problems.
I fully support my peers working on climate science, which is why I joined University Rebellion and Scientist Rebellion.
Organisations
Other contributions
Journal papers:
- Wen*, J., Thibeau-Sutre*, E., DĂaz-Melo, M., Samper-GonzĂĄlez, J., Routier,A., Bottani, S., Dormont, D., Durrleman, S., Burgos, N. and Colliot, O.,âConvolutional Neural Networks for Classification of Alzheimerâs Disease: Overview and Reproducible Evaluationâ, Medical Image Analysis, 63, 101694 (2020) doi:10.1016/j.media.2020.101694 â hal-02562504 (*: joint first authorship)
- Couvy-Duchesne*, B., Faouzi*, J., Martin*, B., Thibeau-Sutre*, E., Wild*, A., Ansart, M., Durrleman, S., Dormont, D., Burgos, N. and Colliot, O., âEnsemble Learning of Convolutional Neural Network, Support Vector Machine, and Best Linear Unbiased Predictor for Brain Age Prediction: ARAMIS Contribution to the Predictive Analytics Competition 2019 Challengeâ, Frontiers in Psychiatry, 11 (2020) doi:10.3389/fpsyt.2020.593336 â hal-03136463 (*: joint first authorship)
- Burgos*, N., Bottani*, S., Faouzi*, J., Thibeau-Sutre*, E. and Colliot, O., âDeep learning for brain disorders: from data processing to disease treatmentâ, Briefings in Bioinformatics, 22(2), 1560â1576 (2021) doi:10.1093/bib/bbaa310 â hal-03070554 (*: joint first authorship)
- Chadebec, C., Thibeau-Sutre,E., Burgos, N. and Allassonnière, S., âData augmentation on neuroimaging data with variational autoencodersâ, IEEE Transactions on Pattern Analysis and Machine Intelligence (2022) 10.1109/TPAMI.2022.3185773 â arXiv: 2105.00026
Journal papers linked to software contributions:
- Routier, A., Burgos, N., Guillon, J., Samper-GonzĂĄlez, J., Wen, J. and Bottani, S.,Marcoux, A., Bacci, M., Fontanella, S., Jacquemont, T., Wild, A., Gori, P., Guyot,A., Lu, P., DĂaz, M., Thibeau-Sutre, E., Moreau, T., Teichmann, M., Habert, M.-O., Durrleman, S. and Colliot, O., âClinica: an open source software platform forreproducible clinical neuroscience studiesâ, Frontiers in Neuroinformatics, 15 (2021) doi:10.3389/fninf.2021.689675 â hal-02308126
- Thibeau-Sutre*, E., DĂaz*, M., Hassanaly, R., Routier, A., Didier, D., Colliot, O.,Burgos, N., âClinicaDL: an open-source deep learning software for reproducibleneuroimaging processingâ, Computer Methods and Programs in Biomedicine (20220 10.1016/j.cmpb.2022.106818 â hal-03351976 (*: joint first authorship)
Peer-reviewed conference proceedings:
- Thibeau-Sutre, E., Colliot, O., Dormont, D. and Burgos, N., âVisualization approach to assess the robustness of neural networks for medical image classificationâ, SPIE Medical Imaging, 11313, 113131J, 2020 doi:10.1117/12.2548952 â hal-02370532
- Thibeau-Sutre, E., Couvy-Duchesne, B., Dormont, D., Colliot, O. and Burgos, N., âMRI Field Strength Predicts Alzheimerâs Disease: a Case Example of Bias in the ADNI Data Setâ, ISBI - International Symposium on Biomedical Imaging, 2022 doi:10.1109/ISBI52829.2022.9761504 â hal-03542213
- Thibeau-Sutre, E., Wolterink, J., Dormont, D., Colliot, O. and Burgos, N., âHow can data augmentation improve attribution maps for disease subtype explainability?â, SPIE Medical Imaging, 2023 hal-0396673
Research profiles
Courses academic year 2022/2023
Courses in the current academic year are added at the moment they are finalised in the Osiris system. Therefore it is possible that the list is not yet complete for the whole academic year.
Current projects
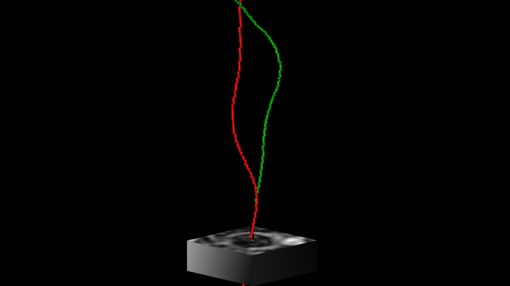
Automatic carotid segmentation
Collaboration with McGill University
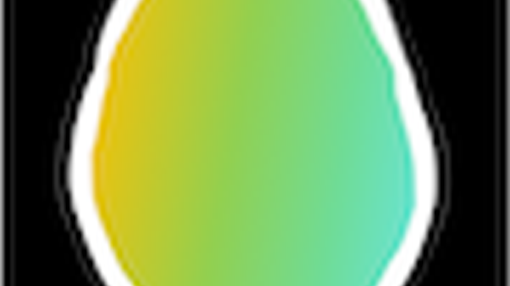
Brain-SHIFT
an innovative AI tool to select Subdural Hematoma Invasive or Follow-up Treatment
The midline shift is a well-known measurement used in the clinical routine to estimate the deformation of the pathological brain, particularly in the case of a chronic subdural hematoma. However, this measurement is local and does not reflect the pressure encountered by all neurological regions. Then there is an unmet need for new measurements considering the deformation of all parts of the brain. The aim of this project is to develop a method to estimate the deformation field encountered by the whole brain when a hematoma is growing in the skull, which is a constrained space. First, anatomical landmarks such as the skull, the midplane of the brain and the hematoma volume will be automatically computed. Then, they will be used by a deep learning network learning to estimate a clinically relevant brain deformation field: a deformation field that allows to estimate a pseudo-healthy scan from the original one. Ultimately this study will allow to develop new measurements that could be used by the clinical team to decide which treatment should be given to patients with chronic subdural hematomas. This project was awarded by the PIHC 2023 fund (60kâŹ)
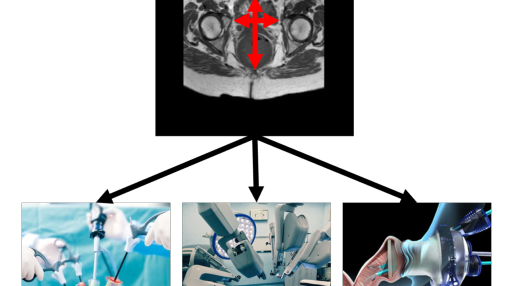
AI-SURGE
Towards personalized AI-based treatment selection in colorectal cancer surgery
The choice of the procedure to be performed in colorectal cancer surgery is crucial, as a wrong choice may lead to poor postoperative outcomes. As it was shown that surgical outcomes may depend on pelvic anatomy, we propose the project AI-surge: a decision support tool for surgeons based on artificial intelligence computing pelvimetry parameters. The booster project will allow to implement the first part of this tool, which is the automatic determination of the pelvis shape from MR images. This project was awarded by the CMI-NEN consortium (15kâŹ).
News on utwente.nl
Events with University Rebellion: