I am a PhD candidate in the section of Industrial Engineering and Business Information Systems (IEBIS) and obtained a master in Industrial Engineering and Management from the University of Twente in 2021.
I work in the general areas of operations management and machine learning. More specifically, my focus is on sequential decision-making under uncertainty for transportation and logistics management problems. My main methodological focus is on reinforcement learning and approximate dynamic programming.
My research involves the development of DynaPlex; an artificial intelligence toolbox containing various (deep) reinforcement learning algorithms that can be applied to a variety of dynamic data-driven logistics challenges. The DynaPlex project is in collaboration with the Eindhoven University of Technology and a wide range of companies.
Expertise
Computer Science
- Reinforcement Learning
- Benchmarking
- World Application
- Vehicle Routing
Economics, Econometrics and Finance
- Learning
- Benefits
- Cost Function
- Regression Model
Organisations
Publications
2024
2023
2022
2020
Research profiles
Courses academic year 2024/2025
Courses in the current academic year are added at the moment they are finalised in the Osiris system. Therefore it is possible that the list is not yet complete for the whole academic year.
Courses academic year 2023/2024
Address
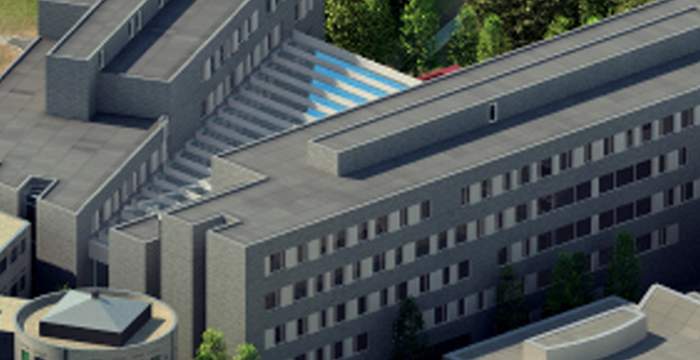
University of Twente
Ravelijn (building no. 10), room 3402
Hallenweg 17
7522 NH Enschede
Netherlands
University of Twente
Ravelijn 3402
P.O. Box 217
7500 AE Enschede
Netherlands