Hao Cheng is an Assistant Professor at the Department of Earth Observation Science at ITC Faculty Geo-Information Science and Earth Observation from the University of Twente, the Netherlands.
Prior to this appointment, he was a researcher and MSCA posdoctoral fellow at the ITC Faculty Geo-Information Science and Earth Observation, University of Twente, the Netherlands, and a posdoctoral researcher at the Institute of Cartography and Geoinformatics, Leibniz University Hannover, Germany.
He earned his M.Sc. degree in Internet Technologies and Information Systems from TU Braunschweig, Leibniz University Hannover, TU Clausthal, and University Göttingen, Germany, in 2017, and his Ph.D. at the Faculty of Civil Engineering and Geodetic Science, Leibniz University Hannover, Germany, 2021.
His research interests include accessible and responsible GeoAI, deep learning of road user behavior modeling in intelligent transport systems and autonomous driving.
Expertise
Computer Science
- Models
- User
- Autonomous Driving
- Deep Learning
- Learning Approach
- Communication
- Lstm
Social Sciences
- Traffic
Organisations
Currently, I am a recipient of Marie SkĆodowska-Curie Actions (MSCA) European Postdoctoral Fellowship with the 101062870 - VeVuSafety project that aims to learn the interactions between vehicles and vulnerable road users to facilitate safer Intelligent Transportation Systems and Autonomous Driving.
My research interest includes deep learning of road user behavior modeling in Intelligent Transport Systems and Autonomous Driving and Safety Analysis between vehicles and vulnerable road users.
Publications
2024
2023
Research profiles
I acquired my M.Sc. degree (with distinction) in Internet Technologies and Information Systems from TU Braunschweig, Leibniz University Hannover, TU Clausthal, and University Göttingen, Germany, in 2017, and Ph.D. (with distinction) at the Faculty of Civil Engineering and Geodetic Science, Leibniz University Hannover, Germany, 2021.Â
Traffic safety is the fundamental criterion for vehicular environments, and for the development of many Artificial Intelligent (AI) systems like self-driving cars and robot navigation systems. Unfortunately, statistics show that direct interactions between vehicles and vulnerable road users (VRUs, e.g., pedestrians and cyclists) still lead to serious injuries and fatalities. From 2010 to 2019 in the EU countries, pedestrians and cyclists account for about 20% and 9% respectively to the annual number of fatalities; Among different types of VRUs, pedestrians are the most vulnerable road users at intersections and require the most protection[2]. For instance, a vehicle driver may fail to recognize an approaching pedestrian at an intersection due to block of views, and some pedestrians may not pay attention to the upcoming traffic while talking to friends or looking at mobile phones. If these dangers are automatically detected in advance, a prompt signal can be sent by a traffic safety alarming system to prevent a potential accident. Moreover, the foreseeable advent of autonomous driving and mobile robot applications highly depends on AI systems that can correctly predict other road users' behaviour, i.e., their intended trajectories in the next seconds. Such systems are required to automatically process the input data extracted from the involved road users and the environment to generate desirable output that represents how they interact and move, to guarantee safe decisions to be made in the following steps.
However, there are some urban environments making detection and prediction of road users' behaviour particularly challenging, e.g., temporarily shared spaces of intersections for vehicle turning or shared spaces as a traffic design[1]. Both types of environments allow direct interactions between vehicles and VRUs with no time or space segregation for different transport modes. Under such weakened traffic rules and due to mutual influence, the road usersâ behaviour tends to be multimodal and stochastic, such as stopping, accelerating, or turning. Often, a road user may have to change direction or travel speed to cope with a sudden change of the motion from others. In such situations, the human road user can subconsciously anticipate others' possible behaviour based on the information of road geometry, transport mode, distance, travel speed, orientation, and even other acoustic and facial information, to adjust her or his own movement accordingly. To the contrary, it is still a big challenge for AI systems to perceive the environment and automatically learn road users' behaviour in such kind of situations, and at the same time to protect road usersâ personal information while processing massive traffic data.
This proposal addresses the question on how to leverage heterogenous traffic data automatically and effectively with a consideration of data protection to learn road users' behaviour in various situations for traffic safety between vehicles and VRUs, especially at places where they confront each other. Â
Address
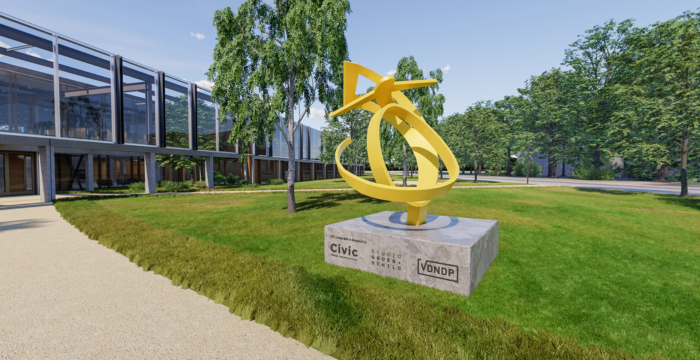
University of Twente
Langezijds (building no. 19), room 1308
Hallenweg 8
7522 NH Enschede
Netherlands
University of Twente
Langezijds 1308
P.O. Box 217
7500 AE Enschede
Netherlands