He received his masters’ degree in computer science at the University of Twente in 1992 and completed his PhD on Formal operation definition in object-oriented databases in 1997. His research targets robustness in data science focusing on two main threats to data science reliability: data quality and undesirable machine learning behaviour. The former is focused on data integration, semi-structured data, natural language processing, and data quality issues involved in these. He co-developed one of the most scalable XML database systems of its time: MonetDB/XQuery. Furthermore, he proposed a data integration approach, called Probabilistic Data Integration, which fundamentally incorporates handling of uncertain and of lesser quality data. He developed a probabilistic database system, called DuBio, which allows the scalable storage, manipulation and management of such uncertain data. On the threat of undesirable machine learning behaviour, he focuses on Explainable AI with the intrinsically explainable deep learning approach ProtoTree as one of the notable results of this. He is secretary of the executive board of the EDBT Association (Extending Database Technology). He is the (co-) author of about 200 publications that accumulated about 2000 citations.
Expertise
Computer Science
- Events
- Database
- Models
- Case Study
- Data Integration
- Real World
- Social Media
- Machine Learning
Organisations
Publications
Research profiles
Affiliated study programs
Courses academic year 2023/2024
Courses in the current academic year are added at the moment they are finalised in the Osiris system. Therefore it is possible that the list is not yet complete for the whole academic year.
- 191211650 - Multi-Disciplinary Design Project
- 192199508 - Research Topics CS
- 192199968 - Internship CS
- 192199978 - Final Project CS
- 192399979 - Final Project BIT
- 201300058 - Research Topics BIT
- 201300059 - Internship BIT
- 201300074 - REDI
- 201300086 - Research Topics 2 CS
- 201400171 - Capita Selecta Software Technology
- 201500371 - Capita Selecta BIT
- 201600017 - Final Project Preparation
- 201600191 - Conference - EngD
- 201700081 - Probabilistic Programming
- 201800524 - Research Topics EIT
- 201900194 - Research Topics I-Tech
- 201900195 - Final Project I-Tech
- 201900200 - Final Project EMSYS
- 201900234 - Internship I-Tech
- 202001022 - Pearls of Computer Science Core
- 202001434 - Internship EMSYS
- 202001486 - Capita Selecta EngD (tailored assignm.)
- 202001521 - Capita Selecta EngD (external course)
- 202001522 - Capita Selecta EngD (in-company tr.)
- 202001613 - MSc Final Project BIT + CS
- 202001614 - MSc Final Project CS + I-Tech
- 202001616 - Research Topics CS + I-TECH
- 202200021 - Machine Learning for Datatypes
- 202200022 - KKR & DQ
- 202200023 - Explainable AI & Ethics
- 202200024 - DS&AI Project
- 202200251 - Capita Selecta DST
- 202200267 - Capita Selecta EngD (tailored assignm.2)
- 202300070 - Final Project EMSYS
- 202300200 - Data Science
- 202300201 - Data Science - Additional Topics
- 202300202 - Data Science for ATLAS
Courses academic year 2022/2023
- 191211650 - Multi-Disciplinary Design Project
- 192166200 - Capita Selecta I-TECH
- 192199508 - Research Topics CS+IST
- 192199968 - Internship CS
- 192199978 - Final Project CS+IST
- 192399979 - Final Project BIT
- 201300058 - Research Topics BIT
- 201300059 - Internship BIT
- 201300074 - REDI
- 201300086 - Research Topics 2 CS+IST
- 201300294 - Master Thesis SEC Computer Science
- 201400171 - Capita Selecta Software Technology
- 201400174 - Data Science
- 201500363 - Data Science Additional Topics
- 201500371 - Capita Selecta BIT
- 201600017 - Final Project Preparation
- 201600191 - Conference - EngD
- 201700081 - Probabilistic Programming
- 201800524 - Research Topics EIT
- 201900194 - Research Topics I-Tech
- 201900195 - Final Project I-Tech
- 201900200 - Final Project EMSYS
- 201900234 - Internship I-Tech
- 202000100 - Introduction to Smart Cities
- 202000101 - Smart City Engineering Project
- 202001022 - Pearls of Computer Science Core
- 202001434 - Internship EMSYS
- 202001486 - Capita Selecta EngD (tailored assignm.)
- 202001521 - Capita Selecta EngD (external course)
- 202001522 - Capita Selecta EngD (in-company tr.)
- 202001613 - MSc Final Project BIT + CS
- 202001614 - MSc Final Project CS + I-Tech
- 202001616 - Research Topics CS + I-TECH
- 202100340 - Combined Final Project EE/S&C
- 202101028 - Data & Information Core
- 202200021 - Machine Learning for Datatypes
- 202200022 - KKR & DQ
- 202200023 - Explainable AI & Ethics
- 202200024 - DS&AI Project
- 202200251 - Capita Selecta DST
- 202200259 - Data & Information Core
- 202200267 - Capita Selecta EngD (tailored assignm.2)
- 202200377 - Internship I-Tech / Robotics
- 202200399 - Internship I-Tech / Robotics
Address
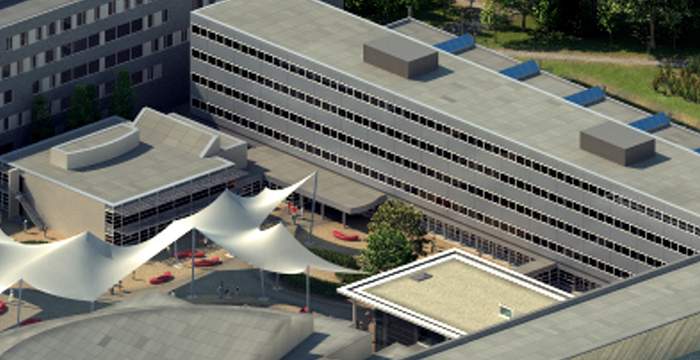
University of Twente
Zilverling (building no. 11), room 4061
Hallenweg 19
7522 NH Enschede
Netherlands
University of Twente
Zilverling 4061
P.O. Box 217
7500 AE Enschede
Netherlands