Rob Bemthuis is an Assistant Professor in the Pervasive Systems Research Group at the University of Twente (UT). He holds both a Master’s and Bachelor’s degree in Industrial Engineering and Management from the UT, with a specialization in production and logistics management. He earned his Ph.D. from the Faculty of Electrical Engineering, Mathematics, and Computer Science (EEMCS) at the same university.
Rob’s research involves IoT- and Big Data-driven solutions to detect and manage emerging behaviors. He was a visiting scholar at the University of Southern Denmark (SDU) and the Karlsruhe Institute of Technology (KIT). Currently, he leads the ECOLOGIC project on sustainable construction logistics. His interests include multi-agent systems, logistics simulation, and applied process mining, with a strong focus on IoT-based approaches.
Alongside his research, Rob actively volunteers at the University of Twente and in Enschede. He served as Treasurer of the PhD Network of the University of Twente (P-NUT) from 2019 to 2020, was a general board member from 2020 to 2021, and then Treasurer and Vice-President from 2021 to 2022. He also chaired the organizing committees of the PhD Day 2019, PhD & PDEng Day 2020, and PhD & PDEng Day 2021.
Expertise
Computer Science
- Process Mining
- Models
- Case Study
- Process Mining Technique
- Process Model
- Simulation Mode
- Events
- Design
Organisations
Publications
2024
2023
Research profiles
My research interests include agent-based modeling, agent-based simulation, multi-agent systems, and process mining, focusing on, but not limited to, the application domains of transportation and logistics, healthcare, as well as construction sites and the built environment.
Selection open bachelor thesis assignments (TCS, BIT and IEM):
Process Mining
- Synthetic Data Generation with LLMs for Process Mining
- Distributed Process Mining
- Various Process Mining Applications
Agent-based Modeling and Simulation (or Multi Agent Systems)
- Extraction of agent models using machine learning
- Extraction of agent models using process mining
- Multi agent system in domain-specific applications
(Co-)Supervision of Bachelor thesis projects (selection):
- Ferdy... (ongoing)
- Ivan... (ongoing)
- Patrick... (ongoing)
- Allard van der Hooft (2024). Development of an intelligent multi-sensor system for real-time monitoring and analysis of soil pollution on construction sites. Study: CreaTe.
- Erjan Steenbergen (2024). Determining Case Identifiers in Event Logs from Hoists in Construction Sites to Estimate the Correlation between Usage Rates and BPMNs. Study: CS.
- Fran Karlović (2024). Developing a Wearable Sensor Network for Air Pollution Monitoring on Construction Sites. Study: CreaTe.
- Hein Huijskes (2024). Effects of Variable Snapshot Frequency on Object Tracking. Study: CS.
- Viktoriia Konashchuk (2024). Towards automated prevention of rework in software development. Study: BIT.
- Xander Küpers (2024). Idle Identification of Construction Machinery through a Deep Learning-Based Algorithm Embedded in Surveillance Camera Systems. Study: CS.
- Denise den Hartog (2022). Event record-based evaluation of business scenarios in the logistics domain using process mining. Study: BIT.
- Floor Rademaker (2022). Subpopulation process comparison for in-hospital treatment processes: a case study for sepsis treatment. Study: BIT.
- Tom Baas (2022). Creating standardized process mining applications based on the open trip model. Study: IEM.
- Venelina Pocheva (2022). Outsourcing Prioritization for Bottleneck Processes Using Process Mining: A Logistics Case Study. Study: CS.
- Dennis Maneschijn (2021). Finding the Appropriate Level of Abstraction for Process Mining in Logistics. Study: BIT.
- Juri van Midden (2021). Using process mining and event log analysis for better business strategy decision-making. Study: BIT.
- Jennifer Cutina (2020). Assessing the use of process mining techniques to monitor the work process of commercial drivers. Study: BIT.
- Julian Stellaard (2020). Tracking the process of an outbreak to a pandemic via logistical infrastructures: case study. Study: CS.
- Niels van Slooten (2020. A maturity level assessment of process mining bottleneck analysis techniques. Study: BIT.
- Simon Arends (2020). Quantity meets quality for mined process models through simulated events. Study: CS.
(Co-)Supervision of Master thesis projects (selection):
- Aletta (ongoing)
- Rinant (ongoing)
- Bas (ongoing)
- Stefan (ongoing)
- Stef Kosters (2024). Development of a Multi-Objective Resource-Constrained Scheduling Method for the Utility Construction Sector. Study: IEM.
- Ruben Govers (2023). An integrated process mining and data mining approach for the validation of agent-based simulation models. Study: BIT.
- Annemiek Fladderak (2022). Developing a planning and control policy for inventory cycle counting by UAVs. Study: IEM.
- Thijs Hammink (2022). Cycle counting with UAVs: Sample selection in time-restricted scenarios with neural network predictions. Study: IEM.
External
- Stockholm University
Supervision of Doctoral candidates
- Fatemeh Massah (EngD, 2024-ongoing)
- Egemen İşgüder (PhD, 2024-ongoing)
- Arda Satici (EngD, 2025-ongoing)
Affiliated study programs
Courses academic year 2024/2025
Courses in the current academic year are added at the moment they are finalised in the Osiris system. Therefore it is possible that the list is not yet complete for the whole academic year.
- 192199508 - Research Topics CS
- 192199968 - Internship CS
- 192199978 - Final Project CS
- 192320111 - Architecture of Information Systems
- 192320501 - Electronic Commerce
- 192320601 - Multi Agent Systems
- 201400171 - Capita Selecta ST
- 201600191 - Conference - EngD
- 201800524 - Research Topics EIT
- 202000591 - IBA/IEM Study Tour Minor
- 202001484 - Architecture of Inform. Systems EngD
- 202001613 - MSc Final Project BIT + CS
- 202100244 - Pervasive Computing
- 202300200 - Data Science
- 202300201 - Data Science - Additional Topics
- 202300202 - Data Science for ATLAS
- 202300241 - Business Intelligence & Databases
- 202400203 - Data Science for GZW
- 202400610 - Process Mining
Courses academic year 2023/2024
Current projects
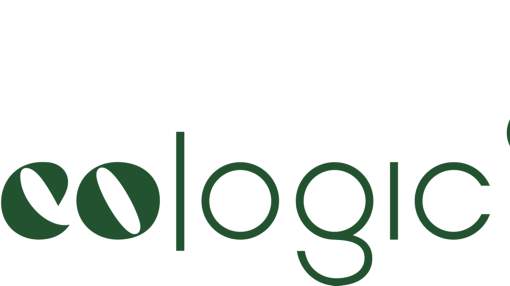
ECOLOGIC
Emission COntrol and Logistics Optimization for Green Infrastructure Construction
The research project ‘ECOLOGIC – Emission Control and Logistics Optimization for Green Infrastructure Construction’ aims to significantly improve the sustainability of the Dutch construction logistics sector by developing reliable data-driven insights and advanced analysis techniques (IoT and AI), through a real-time Carbon Digital Twin (DT), evaluated and demonstrated in two Living Labs. Through anticipatory and adaptive logistics planning, the emission footprint will be minimized while operations are optimized. The approach consists of the "Triple A": Acquire (collect and analyze data), Anticipate (predict), and Adapt (adjust to situations). The principle of federated data sharing is applied, where users only receive feedback on matters they can control themselves.
ECOCHECK
Environmental Construction Observation and Control using High-tech Equipment and Construction Knowledge
The construction industry is in a crisis akin to a "lockdown"?, with projects delayed and deadlines postponed due to the emission crisis. Growing public and regulatory pressures underscore the importance of sustainability in construction. ECOCHECK uses intelligent sensors to unlock real-time data on energy consumption and noise levels at construction sites. This data-driven approach not only optimizes energy use and reduces noise pollution, but also holds the key to lifting the industry out of its current standstill. By checking what happens in reality, rather than relying solely on theoretical models, ECOCHECK ensures we stay on top of these pressing issues.
Finished projects
In the press
- Bitterballentafel: Wat is de waarde van gedeelde data voor het optimaliseren van je planning? (2024)
- Minder schade door logistieke ontwrichting (2023) / Less damage from logistics disruptions (2024)
- Kick-off Onderzoeksproject ECOLOGIC (2023)
- DataREL: De strijd tegen logistieke disrupties (2023) (link2)
- DATAREL: project update (2022)
- “De mensen achter Countdown” – Rob Bemthuis (2021)
- PhD & PDEng Day 2019 - University of Twente (2019)
News on utwente.nl
- Dr.ir. Rob Benthuis defends his PhD (2024)
- ‘I’ve faced the nearly stereotypical PhD experience: mental health challenges’ (2021)
- PhD & PDEng Day explores the role of scientists in ‘post-fact society’ (2021)
- P-NUT: ‘There should be serious consequences to misconduct’ (2021)
- PhD Day: ‘We want to be honest about how things really are' (2019)
- Best Doctoral Consortium Paper Award at the IEEE EDOC 2019 conference (2019)
- Poster nomination for Rob Bemthuis at ICT.Open (2019)
- Commit2Data project, Datarel, at ICT Open 2019 (2019)
- Succes UT-studenten bij Griekse summerschool (2016)
Address
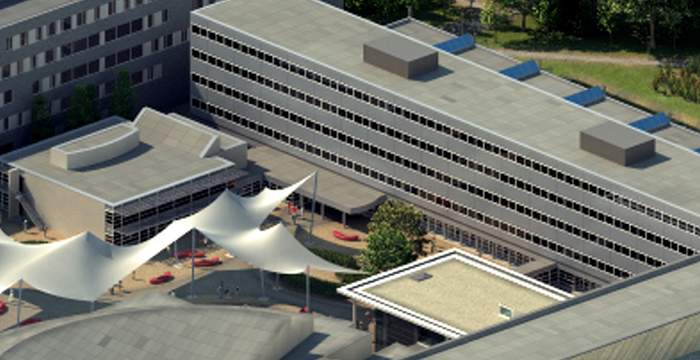
University of Twente
Zilverling (building no. 11), room 5010
Hallenweg 19
7522 NH Enschede
Netherlands
University of Twente
Zilverling 5010
P.O. Box 217
7500 AE Enschede
Netherlands